Warm-Start Reinforcement Learning: From Function Approximation Error to Sub-Optimality Gap
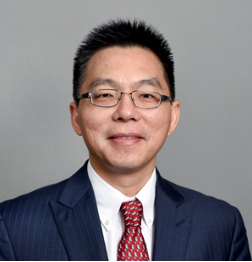
Dr. Junshan Zhang
Professor Department of Electrical and Computer Engineering, UC Davis, Davis
Cerent Engineering Science Complex, Salazar Hall 2009A
4:00 PM
Abstract: Conventional reinforcement learning (RL) techniques face the formidable challenge of high sample complexity and intensive computation load, which hinders RL's applicability in real-world tasks. To tackle this challenge, Warm-Start RL is emerging as a promising new paradigm, with the basic idea being to accelerate online learning by starting with an initial policy trained offline. Indeed, owing to the knowledge transfer from an initial policy, Warm-Start RL has been successfully applied in AlphaZero and ChatGPT, demonstrating its great potential to speed up online learning. Despite these remarkable successes, a fundamental understanding of Warm-Start RL is lacking. The primary objective of this study is to quantify the impact of function approximation errors on the sub-optimality gap for Warm-Start RL. We consider the widely used "Actor-Critic" method for RL. Our findings reveal that a 'good' warm-start policy (obtained by offline training) may be insufficient, and bias reduction in online learning also plays an essential role to lower the suboptimality gap.
Bio: Junshan Zhang is a professor in the ECE Department at University of California Davis. He received his Ph.D. degree from the School of ECE at Purdue University in Aug. 2000, and was on the faculty of the School of ECEE at Arizona State University from 2000 to 2021. His research interests fall in the general field of information networks and data science, including edge AI, reinforcement learning, continual learning, network optimization and control, game theory. He is a Fellow of the IEEE, and a recipient of the ONR Young Investigator Award in 2005 and the NSF CAREER award in 2003. His papers have won a few awards, including the Best Student paper at WiOPT 2018, the Kenneth C. Sevcik Outstanding Student Paper Award of ACM SIGMETRICS/IFIP Performance 2016, the Best Paper Runner-up Award of IEEE INFOCOM 2009 and IEEE INFOCOM 2014, and the Best Paper Award at IEEE ICC 2008 and ICC 2017.