Learning Large-Scale Sparse Graphical Models: Theory, Algorithm, and Applications
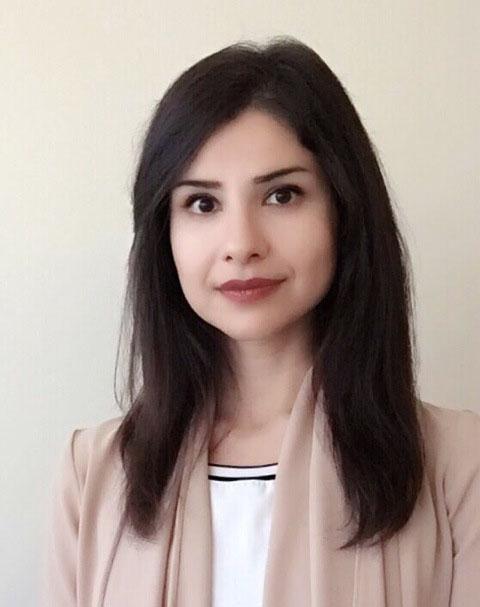
Dr. Somayeh Sojoudi
Assistant Professor EE & CS Department, UC Berkley
Cerent Engineering Science Complex, Salazar Hall 2009A
4:00 PM
Abstract - Learning models from data has a significant impact on many disciplines, including computer vision, medical imaging, social networks and signal processing. In the network inference problem, one may model the relationships between the network components through an underlying inverse covariance matrix. The sparse inverse covariance estimation problem is commonly solved using an ℓ1-regularized Gaussian maximum likelihood estimator, known as “graphical lasso”. Despite the popularity of graphical lasso, its computational cost becomes prohibitive for large data sets. In this talk, we will develop new notions of sign-consistent matrices and inverse-consistent matrices to obtain key properties of graphical lasso and prove that although the complexity of solving graphical lasso is high, the sparsity pattern of its solution has a simple formula if a sparse graphical model is sought. We will prove — under mild assumptions— that the graphical lasso estimator can be retrieved by soft-thresholding the sample covariance matrix and solving a maximum determinant matrix completion (MDMC) problem, and describe a Newton-CG algorithm to efficiently solve the MDMC problem. We will illustrate our results in different case studies.
Dr. Somayeh Sojoudi is an Assistant Professor in the Departments of Electrical Engineering & Computer Sciences and Mechanical Engineering at UC Berkeley. She is an Associate Editor of the journals of IEEE Transactions on Smart Grid, IEEE Access, and Systems & Control Letters. She is a member of the conference editorial board of the IEEE Control Systems Society. She is a recipient of the 2015 INFORMS Optimization Society Prize for Young Researchers and a recipient of the 2016 INFORMS ENRE Energy Best Publication Award. She was a finalist (as advisor) for the Best Student Paper Award at the 2018 American Control Conference and a finalist (as a co-author) for the best student paper award at the 53rd IEEE Conference on Decision and Control 2014.