Infrared Oscilloscopes: Sampling 30-THz Waveforms for Next-Generation Spectroscopy
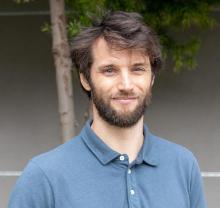
Dr. William Putnam
Assistant Professor
ECE Department, UC Davis, Davis, CA
Thu, 11/17/2022
Abstract: In electronic devices like field-effect transistors, applied electric fields, up to hundreds of gigahertz in frequency, control electron motion. Recently, it has been demonstrated that the electric fields of ultra-intense laser pulses, i.e. electric fields in the tera- to petahertz (THz to PHz) regime, can similarly control electric currents around gas-phase atoms. In this talk, I will describe recent efforts to extend these early demonstrations of THz- and PHz-speed electronics from gaseous media to solid-state, microelectronic devices. Specifically, I will describe nanoantenna-based devices in which electric currents can be switched on and off by individual oscillations of the electric field of an infrared laser pulse. I will show that these devices can be used to sample >30-THz electric field waveforms in the time domain, and I will overview one of the exciting potential applications of these devices: field-resolved infrared spectroscopy.
Bio: William Putnam received his Ph.D. in EECS from MIT as well as undergraduate degrees in EECS and physics. Following his Ph.D. he held postdoctoral positions at MIT and the Center for Free-Electron Laser Science (CFEL) at the University of Hamburg, Germany. After his postdoctoral work and prior to joining the faculty at UC Davis, William spent several years as a staff scientist at Northrop Grumman’s basic research laboratory, NG Next where he worked on ultrafast electronics and frequency comb technology. From his undergraduate years to his postdoctoral work to his time in industry, William’s research has centered around both fundamental and applied studies of optics and quantum electronics.
Learning Preferences for Interactive Autonomy
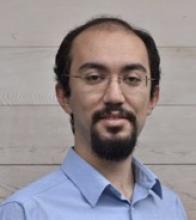
Dr. Erdem Bıyık
Postdoctoral Researcher
Center for Human-Compatible Artificial Intelligence, UC, Berkeley, CA
Thu, 11/03/2022
Abstract: In human-robot interaction or more generally multi-agent systems, we often have decentralized agents that need to perform a task together. In such settings, it is crucial to have the ability to anticipate the actions of other agents. Without this ability, the agents are often doomed to perform very poorly. Humans are usually good at this, and it is mostly because we can have good estimates of what other agents are trying to do. We want to give such an ability to robots through reward learning and partner modeling. In this talk, I am going to talk about active learning approaches to this problem and how we can leverage preference data to learn objectives. I am going to show how preferences can help reward learning in the settings where demonstration data may fail, and how partner-modeling enables decentralized agents to cooperate efficiently.
Bio: Dr. Erdem Bıyık is a postdoctoral researcher at the Center for Human-Compatible Artificial Intelligence at University of California, Berkeley. He has received his B.Sc. degree from Bilkent University, Turkey, in 2017; and Ph.D. degree from Stanford University in 2022. His research interests lie in the intersection of robotics, artificial intelligence, machine learning and game theory. He is interested in enabling robots to actively learn from various forms of human feedback and designing robot policies to improve the efficiency of multi-agent systems both in cooperative and competitive settings. He also worked at Google as a research intern in 2021 where he adapted his active robot learning algorithms to recommender systems. He will join the University of Southern California as an assistant professor in 2023.
Photovoltaic Microinverters and Energy Storage Systems, 2022

Mr. Mark Baldassari
Director of Codes and Standards
Enphase Energy, Petaluma, CA
Thu, 10/20/2022
Abstract: The presentation will cover how solar photovoltaic cells convert solar energy into usable power. The effects on energy production due to insolation (sunlight) and temperature. Simple solar systems cover traditional string inverters versus modern microinverters and discusses the safety benefits. Energy storage is discussed starting with why storage is needed for several applications. A simple single line diagram is presented showing how PV and energy storage can be incorporated into a home. A quiz is presented to test the audience on the major topics presented.
Bio: Mr. Baldassari has over 39 years experience in engineering and product development and over 14 years with Enphase Energy, where he holds the position of Director, Codes and Standards. Currently, he actively participates in several Codes and Standards development groups both internationally and domestically. He is involved with the development of the National Electrical Code, product safety standards with Underwriters Laboratory, and international installation and safety standards. Mr. Baldassari has a bachelor’s degree in electrical and Electronic Engineering from California State University Sacramento.
Class Modular Sensors: Used in Outdoor IoT Monitoring
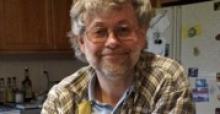
Mr. Neil Hancock
CTO
Azonde, Aznode.com
Thu, 10/06/2022
Abstract: The low-cost Internet of Things architecture is well suited for remote environmental monitoring. This talk covers a device designed to be low cost, solar powered, and used outdoors, for critical stream level monitoring in the drought stricken North Bay. The “open source” Stroud Water Research Center's "Modular Sensors" software and the Mayfly microcomputer facilitate environmental scientists collecting physical measurements from the “great outdoors”. Low-cost board level sensors using ADC, I2C or 1Wire are well understood and relatively low power. External transducers have a wider range of sensing, and require interfaces using the newer USGS SDI-12 three wire water world protocol, and the older stable Modbus 4wire RS485. Each brings new powering challenges. The software code and hardware definitions are stored in git, a distributed “Version Control System” allowing easy incremental traceable improvements. The package comes with technical debt that needs characterizing and analyzing.
Bio: Neil Hancock is a Chartered Engineer, active in circuit design and firmware for 40 years. For the first 20 years he worked with large companies in telecom equipment design and more recently in Internet of Things design for environmental monitoring. Neil volunteers as a Planning Commissioner for the City of Cotati, an Industry Advisor for SSU, is a long-term IEEE member, and enjoys short bicycle rides to coffee shops in Sonoma County. He received his BSc in Electronic Engineering, from City, University of London, United Kingdom.
Chartered Engineers (CEng) develop solutions to engineering problems using new or existing technologies, through innovation, creativity and change and/or they may have technical accountability for complex systems with significant levels of risk.
AI Inference with Intel® FPGA AI Suite
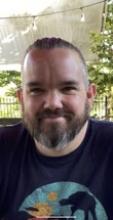
Mr. Kevin Drake
Application Engineer
Intel Corporation
Thu, 09/15/2022
Abstract: Intel® FPGAs enable real-time, low-latency, and low-power deep learning inference combined with the following advantages:
- I/O flexibility
- Reconfiguration
- Ease of integration into custom platforms
- Long lifetime
Intel® FPGA AI Suite was developed with the vision of ease-of-use of artificial intelligence (AI) inference on Intel® FPGAs. The suite enables FPGA designers, machine learning engineers, and software developers to create optimized FPGA AI platforms efficiently.
Utilities in the Intel FPGA AI Suite speed up FPGA development for AI inference using familiar and popular industry frameworks such as TensorFlow* or PyTorch* and OpenVINO toolkit, while also leveraging robust and proven FPGA development flows with the Intel Quartus Prime Software. The Intel® FPGA AI Suite tool flow works with the OpenVINO toolkit, an open-source project to optimize inference on a variety of hardware architectures. The OpenVINO toolkit takes Deep Learning models from all the major Deep Learning frameworks (such as TensorFlow, PyTorch, Keras*) and optimizes them for inference on a variety of hardware architectures, including various CPUs, CPU+GPU, and FPGAs.
Bio: Kevin is an Application Engineer at Intel Corporation. He holds a bachelor’s degree in computer science from Sonoma State University and graduated in 2021. He is currently pursuing his master’s degree in data science at Worcester Polytechnic Institute. At Intel, Kevin works with AI Inference and Acceleration using FPGAs and other ASIC devices. He has also worked in the Intel FPGA University Program to help students learn FPGA design and development in a healthy and equitable environment. Prior to Intel, Kevin was an education and training manager for the US Air Force. During this time, he managed 14 career fields’ training and operational readiness.